CUHK
News Centre
CUHK unveils breakthrough in protein homolog detection with AI technology
The Chinese University of Hong Kong’s (CUHK) Faculty of Engineering has conducted pioneering research in biological sequence analysis to develop an AI-assisted dense homolog retriever (DHR), a groundbreaking method for rapid, sensitive detection of remote protein homologs. This innovative development is a powerful tool for exploring protein evolution, structure and function, which can accelerate drug discovery and clinical therapies. The study results have been published in Nature Biotechnology and highlighted in Nature Methods.
AI-powered DHR: A fast, accurate solution
Protein homolog detection is fundamental for understanding evolutionary relationships and predicting protein functions. However, traditional detection methods, which rely heavily on sequence alignment techniques, such as dynamic programming-based algorithms (e.g. BLAST, FASTA), are time-consuming and often miss distant homologs with low sequence similarity. This limitation hampers the identification of protein networks essential for drug development and functional annotation.
Led by Professor Li Yu, Assistant Professor of the Department of Computer Science and Engineering in the Faculty of Engineering at CUHK, the team has developed DHR, a novel AI-powered method, to resolve the fundamental, decades-old protein homology detection problem in computational biology. Unlike traditional methods, DHR uses dense retrieval, a bi-encoder model and contrastive learning to identify subtle homological relationships. By encoding sequences into dense vectors and using a dot product for scoring, DHR surpasses traditional approaches in both speed and precision. “DHR is particularly adept at discovering distant homologs, which are often missed by traditional methods,” said Professor Yu. “It’s not only faster but also more precise, making it ideal for exploring protein sequence diversity across large databases.”
In an era with more frequent AI applications, as large language models have made contextual retrieval possible, the research demonstrated that DHR has already implemented a similar retriever in the protein homolog search field with promising results. This project was initialised to speed up the homolog search speed of the previous AI model AlphaFold 2, which made use of traditional methods and has been successfully integrated into the researchers’ method in the pipeline, resulting in speeds that are several times faster than before and getting rid of the bottleneck. The team plans to create an open-source and user-friendly tool that will allow broader adoption and continued innovation in protein analysis.
DHR helps with development of targeted therapies and new antibiotics
DHR’s ability to detect distant homologs opens new opportunities in drug discovery. In cancer research, for example, it can identify homologs of proteins involved in cell growth and apoptosis, aiding the development of therapies that target tumor growth or induce cancer cell death.
The tool is also valuable in the fight against antibiotic resistance. By detecting conserved proteins essential for bacterial survival across resistant strains, DHR could help researchers develop new antibiotics that remain effective even as bacteria evolve.
Neurodegenerative diseases such as Alzheimer’s and Parkinson’s involve the misfolding and aggregation of specific proteins. By detecting homologs of these proteins, DHR can contribute to a better understanding of the molecular underpinnings of these conditions. This knowledge may facilitate new treatments that prevent protein misfolding or remove toxic aggregates, potentially slowing disease progression.
The full research paper can be found at: https://www.nature.com/articles/s41587-024-02353-6
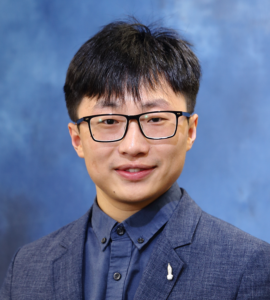
Professor Li Yu, Assistant Professor, Department of Computer Science and Engineering, Faculty of Engineering, CUHK
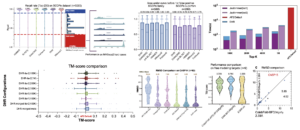
Summary of DHR Results: Sensitivity on the SCOPe dataset, including superfamily-level outcomes; average runtime performance compared with JackHMMER on the CASP dataset, highlighting efficiency improvements; and structure prediction results using MSAs built by DHR compared to default AlphaFold2 results, demonstrating enhanced prediction accuracy.
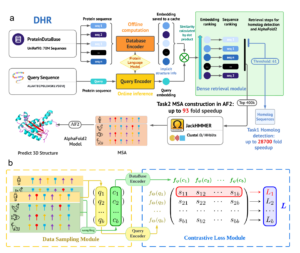
Overview of the DHR Framework: A three-level hierarchical structure focuses on training the protein database encoder and query encoder. Offline inference on the protein database with cached embeddings enables efficient vector-level similarity calculations for ranking sequences. The inference pipeline retrieves the top K most related sequences using dot products. JackHMMER is then applied to this subset to construct an MSA for downstream tasks like 3D structure prediction or protein function forecasting. UniRef90 can be encoded into vectors offline without affecting MSA construction speed.